tech
Challenges in Processing Big Data
The era of big data has revolutionized the way businesses operate, offering valuable insights that drive innovation and informed decision-making. However, with the exponential growth of data, processing and managing it efficiently has become a formidable challenge. In this article, we will explore the myriad challenges in processing big data and delve into expert solutions that address these obstacles effectively.
Challenges in Processing Big Data
1. Volume Overload: Managing the Data Deluge
Processing big data starts with addressing its sheer volume. The exponential increase in data creation, generated by various sources, has overwhelmed traditional data storage and processing methods. The data deluge necessitates scalable and cost-effective solutions.
To tackle this challenge, organizations are embracing distributed storage and processing frameworks like Hadoop and Apache Spark. These tools enable the distributed processing of data across clusters of computers, allowing businesses to handle enormous volumes efficiently.
2. Velocity: Dealing with Real-time Data Streams
In today’s fast-paced world, many applications require real-time data processing. From financial markets to social media platforms, processing data in real-time is essential for making instant decisions and staying ahead of the competition.
To address velocity challenges, technologies like Apache Kafka are gaining prominence. Kafka enables the ingestion and processing of real-time data streams, allowing organizations to act promptly based on the latest information.
3. Variety: Integrating Diverse Data Sources
Big data comes in various formats, including structured, semi-structured, and unstructured data. Integrating and processing data from disparate sources with different formats and structures poses a significant challenge.
Data lakes and data warehouses have emerged as potential solutions to manage data variety. These centralized repositories allow businesses to store diverse data types and run analytics across multiple sources effectively.
4. Veracity: Ensuring Data Quality and Accuracy
The veracity of big data refers to the quality and reliability of the data. With the vast amount of data being generated, ensuring data accuracy and integrity is critical for making sound decisions.
Data cleansing and data quality tools play a crucial role in addressing this challenge. These tools identify and rectify errors, inconsistencies, and inaccuracies, ensuring the data remains reliable and trustworthy.
5. Variability: Handling Seasonal and Unpredictable Data Patterns
Big data exhibits variability due to seasonal patterns, trends, and other unpredictable factors. Analyzing and processing data that follows irregular patterns can lead to inaccurate results.
Advanced statistical models and machine learning algorithms can help tackle data variability. These techniques can identify underlying patterns and trends, making data processing more accurate and insightful.
6. Value Extraction: Turning Data into Actionable Insights
The ultimate goal of processing big data is to extract actionable insights that drive business growth. However, uncovering valuable information from vast datasets is a complex task.
Data visualization and business intelligence tools are essential in extracting actionable insights from big data. These tools present data in a visually appealing manner, enabling decision-makers to grasp trends and patterns easily.
FAQs about Challenges in Processing Big Data
Q: What is the most significant challenge in processing big data?
The most significant challenge in processing big data is managing its sheer volume. The exponential growth of data from diverse sources has overwhelmed traditional storage and processing methods.
A: How can businesses handle the velocity of big data?
Businesses can handle the velocity of big data by adopting real-time data processing technologies like Apache Kafka. This allows them to ingest and process data streams in real-time, enabling prompt decision-making.
Q: How can organizations integrate diverse data sources?
Integrating diverse data sources can be achieved through data lakes and data warehouses. These centralized repositories accommodate various data formats, making it easier to process and analyze the data effectively.
A: What is the importance of data quality in big data processing?
Data quality is paramount in big data processing as it ensures the reliability and accuracy of the insights derived from the data. Data cleansing and quality tools help maintain the integrity of the data.
Q: How can businesses handle seasonal and unpredictable data patterns?
Businesses can handle seasonal and unpredictable data patterns by employing advanced statistical models and machine learning algorithms. These techniques identify underlying patterns, leading to more accurate data analysis.
A: How can organizations extract value from big data?
Organizations can extract value from big data by utilizing data visualization and business intelligence tools. These tools present data in a visually appealing manner, enabling decision-makers to derive actionable insights.
Conclusion
The challenges in processing big data are multifaceted, ranging from handling the overwhelming volume to extracting valuable insights. However, with the right technologies and methodologies, businesses can overcome these obstacles and unlock the true potential of big data. Embracing scalable storage solutions, real-time data processing, and data quality measures are key to thriving in the age of big data. By addressing these challenges head-on, organizations can leverage data-driven insights to stay competitive and drive innovation in their respective industries.
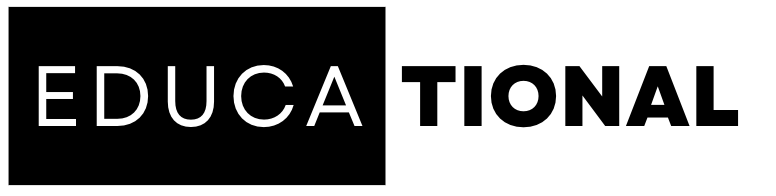