tech
Big Data vs. Traditional Data Analysis
Data has become an invaluable asset, powering various industries and driving decisions across the globe. Organizations have recognized the importance of analyzing data to derive meaningful insights and make informed choices. This has led to the rise of two prominent data analysis approaches: Big Data and Traditional Data Analysis. Both have unique characteristics and play pivotal roles in managing and interpreting data. In this article, we delve deep into the realms of Big Data and Traditional Data Analysis, exploring their fundamental differences, applications, and potential advantages.
Big Data vs. Traditional Data Analysis: An Overview
Big Data: Unraveling the Vast Dimensions
Big Data refers to massive volumes of data, both structured and unstructured, that inundate organizations on a daily basis. The three primary characteristics of Big Data, known as the three V’s, are Volume, Variety, and Velocity. The sheer size of Big Data sets it apart from conventional data sources, making it challenging to manage and analyze using traditional methods.
Traditional Data Analysis: The Conventional Approach
Traditional Data Analysis, on the other hand, revolves around handling moderate-sized datasets using traditional statistical methods. This approach has been the foundation of data analysis for decades and has provided valuable insights across various fields. It relies on structured and organized data, enabling analysts to draw conclusions based on well-established statistical techniques.
The Key Differences
Data Volume: How Much is Too Much?
Big Data: The defining aspect of Big Data is its sheer volume, which ranges from terabytes to petabytes and beyond. This abundance of data requires advanced technologies and tools capable of processing and storing such enormous datasets efficiently.
Traditional Data Analysis: In contrast, Traditional Data Analysis deals with datasets of a more manageable size, typically ranging from kilobytes to gigabytes. This allows analysts to work with data using conventional hardware and software.
Data Variety: Embracing Diversity
Big Data: Apart from its vast volume, Big Data encompasses a wide variety of data types, including structured, semi-structured, and unstructured data. This diversity poses challenges for conventional data analysis tools, demanding innovative approaches to extract insights effectively.
Traditional Data Analysis: Conventional methods primarily deal with structured data, such as relational databases and spreadsheets. While they may handle some forms of semi-structured data, unstructured data presents significant limitations in the traditional context.
Data Velocity: The Need for Speed
Big Data: The velocity of data flow in the Big Data realm is astonishing, with real-time or near-real-time data streaming becoming the norm. Processing and analyzing data at such speeds necessitate specialized tools and technologies that can keep up with the rapid data influx.
Traditional Data Analysis: Traditional approaches often analyze data in batches, which may be suitable for slower data streams. However, real-time analysis of high-velocity data poses challenges to the traditional methods, making it less ideal for certain applications.
Data Veracity: Ensuring Accuracy and Reliability
Big Data: With the massive volume and variety of data, ensuring data veracity becomes critical in the Big Data landscape. Veracity refers to the trustworthiness and reliability of the data, and managing this aspect requires sophisticated data governance and quality control mechanisms.
Traditional Data Analysis: Traditional methods often deal with relatively smaller datasets, making it easier to maintain data veracity. The emphasis on data quality remains, but the scale is smaller, allowing for more stringent control measures.
Data Value: Extracting Actionable Insights
Big Data: Big Data holds immense potential to unlock valuable insights, patterns, and trends hidden within the vast sea of information. Organizations can make data-driven decisions, identify opportunities, and gain a competitive edge by harnessing Big Data analytics.
Traditional Data Analysis: While traditional methods provide valuable insights, their scope might be limited compared to Big Data. The focus is often on generating precise and reliable conclusions rather than exploring extensive datasets.
Applications of Big Data and Traditional Data Analysis
Big Data Applications
- E-Commerce Personalization: E-commerce platforms leverage Big Data to offer personalized product recommendations and tailored shopping experiences.
- Healthcare Insights: Big Data analytics enable the analysis of medical records, aiding in disease diagnosis, treatment optimization, and population health management.
- Social Media Analytics: Social media platforms use Big Data to understand user behavior, improve content recommendations, and enhance engagement.
- Financial Fraud Detection: Financial institutions utilize Big Data analytics to detect fraudulent transactions and enhance security measures.
- Predictive Maintenance: Industries employ Big Data to predict equipment failures, optimize maintenance schedules, and reduce downtime.
- Smart City Initiatives: Big Data facilitates urban planning, traffic management, and resource allocation in smart cities.
Traditional Data Analysis Applications
- A/B Testing: In marketing and web development, traditional data analysis is used for A/B testing to compare the performance of two different versions of a product or service.
- Quality Control: Industries apply traditional data analysis to ensure product quality by analyzing samples and making statistical inferences.
- Survey Analysis: Traditional data analysis techniques are employed to analyze survey responses and draw meaningful conclusions.
- Financial Risk Assessment: Financial institutions use traditional data analysis to assess credit risks and make lending decisions.
- Medical Trials: Traditional data analysis plays a significant role in analyzing data from medical trials to determine the effectiveness of new treatments.
- Sales Forecasting: Businesses use traditional data analysis to forecast sales trends and make informed production and inventory decisions.
The Advantages of Big Data and Traditional Data Analysis
Advantages of Big Data
- Enhanced Decision-Making: Big Data enables data-driven decision-making, leading to more informed and strategic choices.
- Discovering Hidden Patterns: Analyzing vast and diverse datasets allows the identification of patterns that may not be apparent in smaller datasets.
- Real-Time Insights: Big Data analytics can provide real-time or near-real-time insights, facilitating proactive responses to emerging trends.
- Scalability: Big Data technologies offer scalability, allowing organizations to process and store data as their needs grow.
- Competitive Edge: Harnessing Big Data can provide a competitive advantage by identifying unique market opportunities.
Advantages of Traditional Data Analysis
- Statistical Rigor: Traditional data analysis relies on well-established statistical methods, ensuring robustness and reliability in the findings.
- Faster Processing: Smaller datasets in traditional analysis allow for faster processing times compared to Big Data.
- Resource Efficiency: Traditional methods may require less investment in specialized tools and infrastructure, making them cost-effective.
- Clear Interpretations: The simplicity of traditional analysis techniques often results in clearer and more straightforward interpretations.
FAQs
- What is the main difference between Big Data and Traditional Data Analysis? Big Data deals with vast and diverse datasets, while Traditional Data Analysis focuses on smaller, structured datasets, utilizing conventional statistical methods.
- How does Big Data benefit businesses? Big Data helps businesses make data-driven decisions, discover hidden patterns, and gain a competitive edge in their respective industries.
- Can Traditional Data Analysis handle unstructured data? Traditional methods are more suitable for structured data, and while they can handle some semi-structured data, dealing with unstructured data poses challenges.
- What technologies are used for Big Data processing? Technologies like Hadoop, Spark, and NoSQL databases are commonly used for processing and analyzing Big Data.
- What role does data veracity play in Big Data analytics? Data veracity ensures the accuracy and reliability of Big Data, requiring sophisticated data quality control mechanisms.
- Which approach is better for real-time data analysis: Big Data or Traditional Data Analysis? Big Data is more suitable for real-time or near-real-time data analysis due to its ability to handle high-velocity data streams.
Conclusion
In conclusion, the comparison between Big Data and Traditional Data Analysis showcases the significance of both methodologies in today’s data-driven world. While Big Data offers unprecedented opportunities to glean valuable insights from massive and diverse datasets, Traditional Data Analysis continues to play a vital role in handling structured data efficiently. Understanding the unique strengths and limitations of each approach allows organizations to leverage the power of data effectively to make informed decisions and thrive in their respective domains.
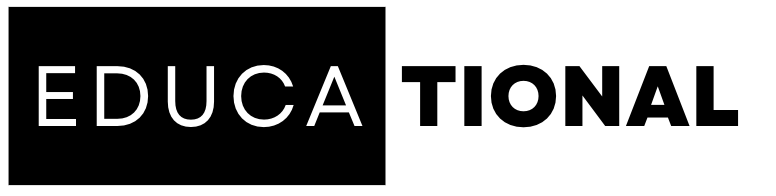